Create a free profile to get unlimited access to exclusive videos, sweepstakes, and more!
Even robots can be fooled, but they're getting smarter
Isn't AI supposed to be programmed for perfection?
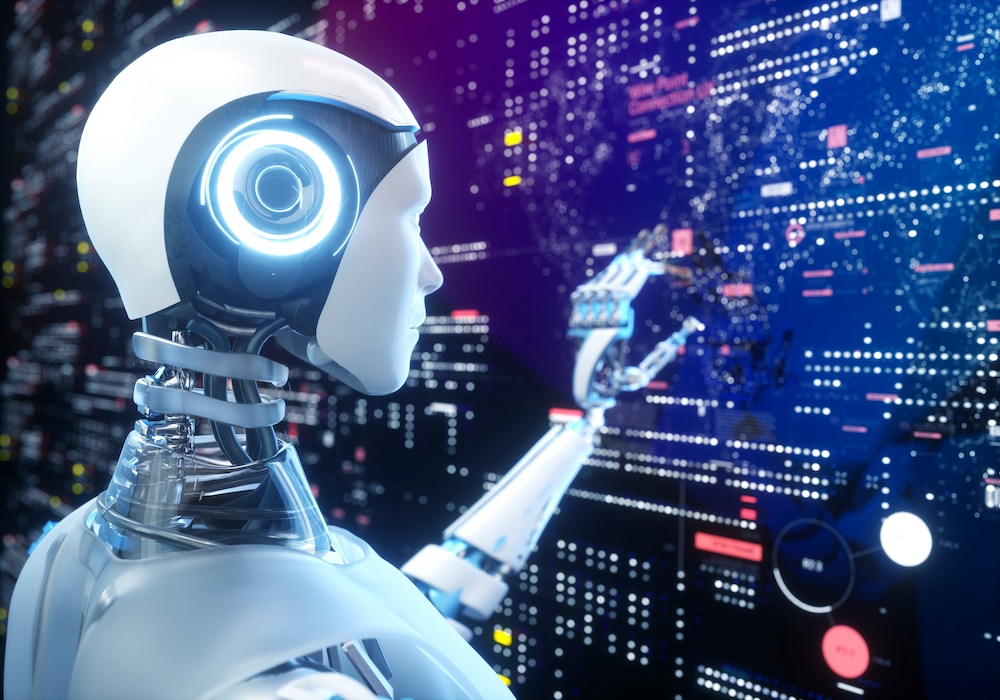
Humans tend to think AI can make no mistakes. Isn’t it programmed to be perfect, something we, as biological organisms, can never be?
Not exactly (and remember that those biological organisms created robots in the first place). If something — a perturbation — gets in the way of the thinking of an artificial brain, it can be deceived. This doesn’t sound like much of a big deal until you realize that just one glitch could mean disaster, depending what the robot is supposed to be in charge of. You can’t have AI getting a dose of medication wrong or telling a system to detonate rather than abort.
Turns out there is still some perfecting to go before robots are infallible. Researcher Jon Vadillo of the University of the Basque Country in Spain has found that AI doesn’t have auditory perception as accurate as that of humans. He and colleague Roberto Santana coauthored a study recently published in Computers & Security, and are now working on reprogramming robots so that they can interpret the signals they hear more accurately.
“[AI] models can be fooled by adversarial examples, which are inputs intentionally perturbed to produce a wrong prediction without the changes being noticeable to humans,” the researchers said in the study.
Just a slight alteration in a signal could mean a completely different interpretation. For example, if someone says “yes” but a perturbation makes the robot hear it the wrong way, it could hear anything from the opposite to something completely unrelated. Imagine saying “yes” to a robot that asks you if your dosage of meds is right and what it hears is instead a command to open the door. It makes no sense, but perturbations can do that. Vadillo and Santanta needed to create perturbations if they were going to find out how they could possibly prevent or reverse them.
Some critical research was already missing. There was no shortage of techniques to create these glitches with, but not much data on what makes them detectable or undetectable to humans. Though there are mind-controlled robots coming into being, human interaction with AI usually means there is some form of speech involved. AI can get away with a perturbation if the human on the other side can’t detect it. An experiment was designed to test human perception of perturbations, and found that previous methods that were thought to be foolproof may not be.
Perturbations can be individual or universal. Individual types are designed for only one particular type of input, like the word “yes.” These are unlikely to confuse the AI when applied to anything else. Universal perturbations are the ones you really have to watch out for, because the specific input doesn’t matter, so they can twist an AI many different ways. They can also pull off these attacks without having to generate a different individual perturbation. Some work only for certain groups of inputs, and these were the type Vadillo and Santana focused on.
What the researchers realized was that their perturbations, despite being deemed acceptable in most AI evaluations, were easily detected by humans. The subjects were able to pick them out as artificial. How effective perturbations were in throwing off an AI also depended on how much they were distorted, and how that distortion is perceived depending on what they heard. A “yes” that was only slightly mispronounced would have a different effect than a hardly recognizable “yes.” Upgrading the detectability of perturbations will make this less of a problem.
“[Our results] stress the need to include human evaluation as a necessary step for validating methods used to generate adversarial perturbation in the audio domain,” the researchers said. “We hope that future works could advance in this direction in order to fairly evaluate the risk that adversarial examples suppose.”